Using artificial intelligence to detect freezing of gait in Parkinson’s patients with wearable devices
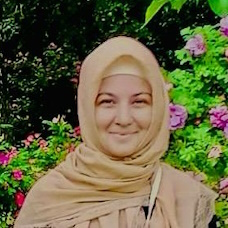
Artificial intelligence (AI) has become an integral part of our daily lives, getting into almost every field imaginable. While some of us stay passive to its extensive presence, others celebrate its advancements with excitement. However, a significant number of people see AI as a potential threat. Whether AI will eventually pose a true danger or remain a beneficial tool, constrained by human-written code, is a question only time can answer.
In this post, I will explore how artificial intelligence can improve the medical field, especially in the identification of Parkinson’s disease. This neurodegenerative illness, which causes motor symptoms like tremors and gait difficulties, affects millions of people around the world. Early and accurate detection is critical for effective therapy and increasing patients’ quality of life. Artificial intelligence showed amazing promise in recognizing subtle patterns and signs of Parkinson’s disease that traditional diagnostic approaches may miss.
Join me as we explore how artificial intelligence is changing Parkinson’s disease detection, obtaining incredible results and bringing hope for a future in which technology significantly improves medical diagnosis and treatment.
The World Health Organization reports that the global incidence of Parkinson’s disease (PD) has doubled in the previous 25 years. Unfortunately, PD affects 7 to 10 million people worldwide, according to estimates also, PD-related disability and mortality rates are increasing faster than those for other neurological disorders and PD accounted for 5.8 million disability-adjusted life years, an 81% rise since 2000, and 329,000 fatalities, a more than 100% increase. Moreover, PD, the second most common neurodegenerative illness, causes specific motor symptoms such as resting tremors, trunk and limb rigidity. One especially unpleasant symptom is freezing of gait (FOG), in which patients feel as if their feet are stuck to the ground, making it impossible to begin or continue walking or turning. Detecting and monitoring FOG is critical for optimizing treatment plans and increasing Parkinson’s patients’ quality of life.
Challenges in FOG Detection
FOG’s intermittent and unexpected character makes it difficult to identify and measure using conventional clinical approaches. Healthcare practitioners frequently depend on patient reports and observations during clinical visits, which may not accurately reflect the full scope of FOG events noticed by patients in their everyday lives. Manual monitoring is additionally vulnerable to human mistakes.
The Role of Wearable Devices and AI
Wearable devices, such as 3D accelerometers worn on the back, provide continuous, objective assessments of a patient’s movement patterns. These sensors collect extensive data on acceleration in various directions, which can be used to identify small changes related with FOG episodes. AI techniques, particularly machine learning models, may be taught on accelerometer data to identify distinct FOG patterns.
Data collection and preprocessing
The initial stage is to collect motion data using wearable sensors worn by Parkinson’s sufferers during regular activities. Accelerometer data is acquired often and requires preprocessing to reduce noise, errors, and extract important characteristics that define FOG events. Data preparation is critical for ensuring the correctness and dependability of the AI model.
Machine Learning Model Development
Several machine learning algorithms may be used for FOG detection, including:
Deep Learning Models: Deep learning is a subset of AI that uses neural networks with many layers (hence ”deep”) to analyze data. Convolutional Neural Networks (CNNs) and Long Short-Term Memory (LSTM) networks are types of deep learning models that can detect complex patterns in time-series accelerometer data.
Machine Learning Models: Machine learning is an umbrella term that includes deep learning and other algorithms. Random forests, for example, are ensemble learning systems that use several decision trees to increase prediction accuracy. These models excel at handling high-dimensional data and nonlinear relationships, making them suitable for FOG detection.
These models do well in understanding the smallest nuances in movement data, making them ideal for detecting the minor clues that follow FOG episodes.
The AI models are trained on labelled datasets containing FOG episodes observed through clinical evaluations or patient self-reports. Learning from this data allows the models to distinguish different movement patterns that occur before and during FOG episodes.
The effectiveness of AI models in detecting FOG is evaluated using several metrics:
- Accuracy: The proportion of correctly identified instances (both FOG and non-FOG) out of all instances.
- Sensitivity: The model’s ability to correctly identify true FOG events.
- Specificity: The model’s ability to correctly identify non-FOG events.
Clinical Applications and Impact
AI-enabled FOG detection may change Parkinson’s care by providing real-time monitoring and notifications to patients and caregivers when FOG episodes occur. Therefore, the number of injuries caused by falls may be decreased.
Remote monitoring allows for less frequent medical visits. Thus, financial savings can be made in the health industry. Furthermore, patients and their families save themselves from the problems and distress that come with unnecessary hospital visits.
Also, optimizing treatment techniques using objective, continuous data and advancing early intervention and tailored treatment for Parkinson’s patients are advantages of these AI techniques.
Conclusion & Future Directions and Challenges
In conclusion, AI-driven FOG detection via wearable devices provides a game-changing approach to Parkinson’s disease management. Healthcare professionals can enhance patient outcomes and quality of life for people with Parkinson’s disease by using the potential of artificial intelligence and continuous monitoring. Further technological breakthroughs and collaboration among physicians, biomedical experts, AI researchers, and industry partners will accelerate the use of AI solutions for FOG detection in clinical practice
Future research aims to create wearable devices that are reliable and easy to use for long-term FOG monitoring, integrate AI models with smartphone applications or smartwatches to enable continuous data collection and analysis, and address issues of model interpretability, ethical considerations, and data privacy.
AI can be used effectively in medical fields and other areas that benefit humanity, and maybe it is not an enemy for humans.
Ayse Kosal Bulbul
I am a doctoral researcher in the clinical research programme, studying the application of artificial intelligence to improve healthcare outcomes. My work focuses on using advanced AI techniques to enhance the detection, diagnosis, and treatment of various medical conditions.
Doctoral Researcher in Clinical Research Programme and Project Researcher in AI4HOPE project.
Institute of Biomedicine, Faculty of Medicine,
University of Turku,
References
[1] World Health Organization. Neurological Disorders: Public Health Challenges, Scitech Book News; World Health Organizatio: Geneva, Switzerland, 2007; p. 31.
[2] S. Sveinbjornsdottir, ‘The clinical symptoms of Parkinson’s disease’, J. Neurochem., vol. 139, no. S1, pp. 318–324, Oct. 2016, doi: 10.1111/jnc.13691.
[3] A. Reeve, E. Simcox, and D. Turnbull, ‘Ageing and Parkinson’s disease: why is advancing age the biggest risk factor?’, Ageing Res. Rev., vol. 14, pp. 19–30, 2014.